研究概要
予測符号化理論は、脳は感覚情報を複数の抽象化レベルで連続的に予測しながら更新し、予測と実際の感覚入力が異なる場合に予測誤差信号を発する、と提案しています。この理論は、知覚、行動、そして創造性などの内部プロセスを理解するための包括的な枠組みを提供するものです。現在、私たちは、以下のテーマに着目して研究を進めています。
予測符号化回路:異なる階層レベルおよび感覚領域における予測符号化に不可欠な微小回路および巨大回路の複雑なネットワークを解明することを目標としています。さまざまな種(ネズミ、マーモセット、ヒト等)を対象とした実験結果と計算モデリングを組み合わせて包括的な洞察を得ます。
予測関連精神疾患の神経マーカー:私たちの研究の重要な焦点は、予測障害を特徴とする精神障害(自閉症スペクトラム障害など)と関連する機構的神経マーカーを特定することです。このような神経基盤を理解することで、障害をよりよく理解し、高解像度の診断と標的を定めた介入に繋げることができると考えられます。
創造性における予測符号化:創造性ポテンシャル、すなわち今後のタスクに対して新規かつ有用なアイデアを生成する脳の能力における予測符号化の役割を探求しています。この複雑なプロセスの仕組みについての機構的な知見を得、創造性を神経回路実装に直接リンクすることを目標にしています。
実用的なアプリケーションに向けた創造性増強:野心的なテーマとして、実世界のアプリケーションで創造性ポテンシャルを強化するクローズドループシステムの開発も目指しています。このシステムは、イノベーションを促進するような革新的なツールと方法論を提供し、創造的な問題解決が求められる分野に変革を起こす可能性があります。
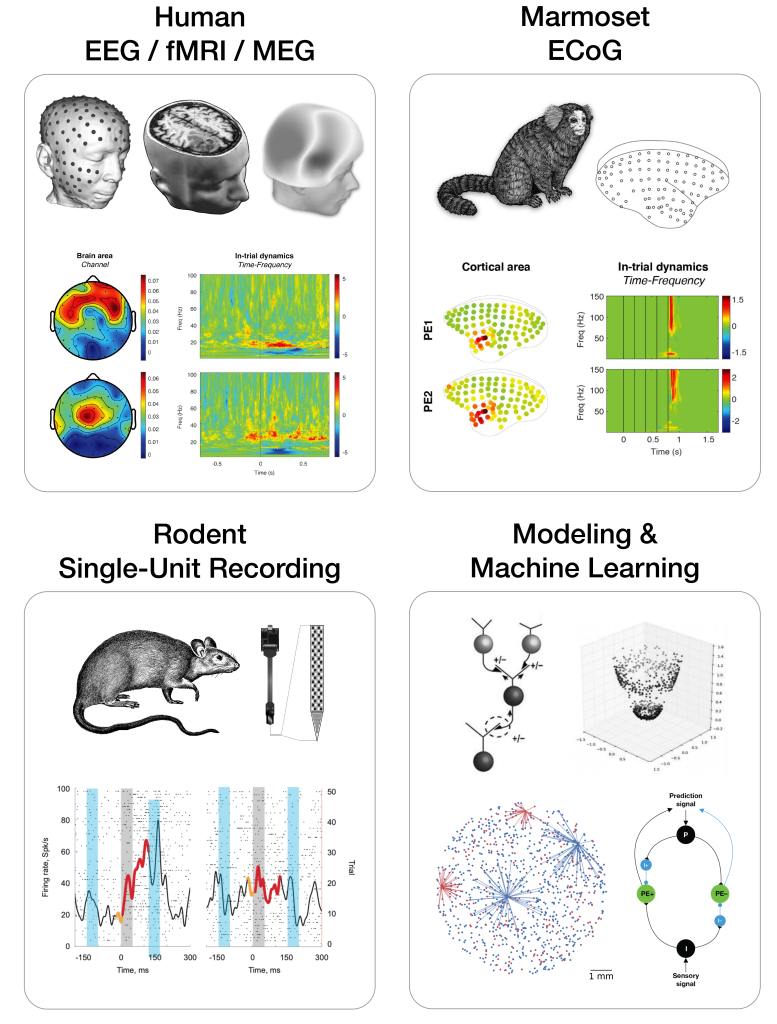
主要論文
Chao ZC, Komatsu M, Matsumoto M, Iijima K, Nakagaki K, Ichinohe N (2024). Diverse configurations of erroneous predictive coding across brain hierarchies in a non-human primate model of autism spectrum disorder. Communications Biology, 7(1), 851.
Huang YT, Wu CT, Fang YM, Fu CK, Koike S, Chao ZC (2024). Crossmodal hierarchical predictive coding for audiovisual sequences in human brain. Communications Biology, 7(1), 965.
Chao ZC, Komatsu M, Matsumoto M, Iijima K, Nakagaki K, Ichinohe N (2024). Diverse configurations of erroneous predictive coding across brain hierarchies in a non-human primate model of autism spectrum disorder. Communications Biology, 7, 851.
Kern FB, Chao ZC (2023). Short-term neuronal and synaptic plasticity act in synergy for deviance detection in spiking networks. PLOS Computational Biology, 19(10), e1011554.
Chao ZC, Huang YT, Wu CT (2022). A quantitative model reveals a frequency ordering of prediction and prediction-error signals in the human brain. Communications Biology, 5(1), 1076.
Chao ZC, Takaura K, Wang L, Fujii N, Dehaene S (2018). Large-scale cortical networks for hierarchical prediction and prediction error in the primate brain. Neuron, 100(5), 1252-1266.
Chao ZC, Nagasaka Y, Fujii N (2015). Cortical network architecture for context processing in primate brain. Elife, 4, e06121.
略歴
私は人間の心とは何かということにずっと興味を抱いてきました。人間に近い知性を持つマシンが実現するならば、そこに自由な意思や意識は宿るのでしょうか。私は、台湾の大学で生命科学ならびに化学を専攻し、その後米国ジョージア工科大学の博士課程において医用生体工学を学びました。博士論文では、ペトリ皿で培養した神経細胞をロボットに接続し、そのロボットが目的に適った行動を習得できることを実証しました。学位取得後に来日し、理化学研究所脳科学総合研究センター(BSI)にて研究員、国立生理学研究所にて助教、そして京都大学にて講師を務め、ヒトとサルの行動から脳シグナルを紐解き、脳がどのようにロボットやコンピュータを制御するのかを理解することに努めてきました。そして2019年9月、このIRCNに特任准教授として着任しました。ここでは、これまでに得たコンピューティング系実験スキル、培養系実験スキル、生体系実験スキルを活かし、「認知理論の統一的見解」をもたらす予測コーディングのエビデンスを探求したいと考えています。