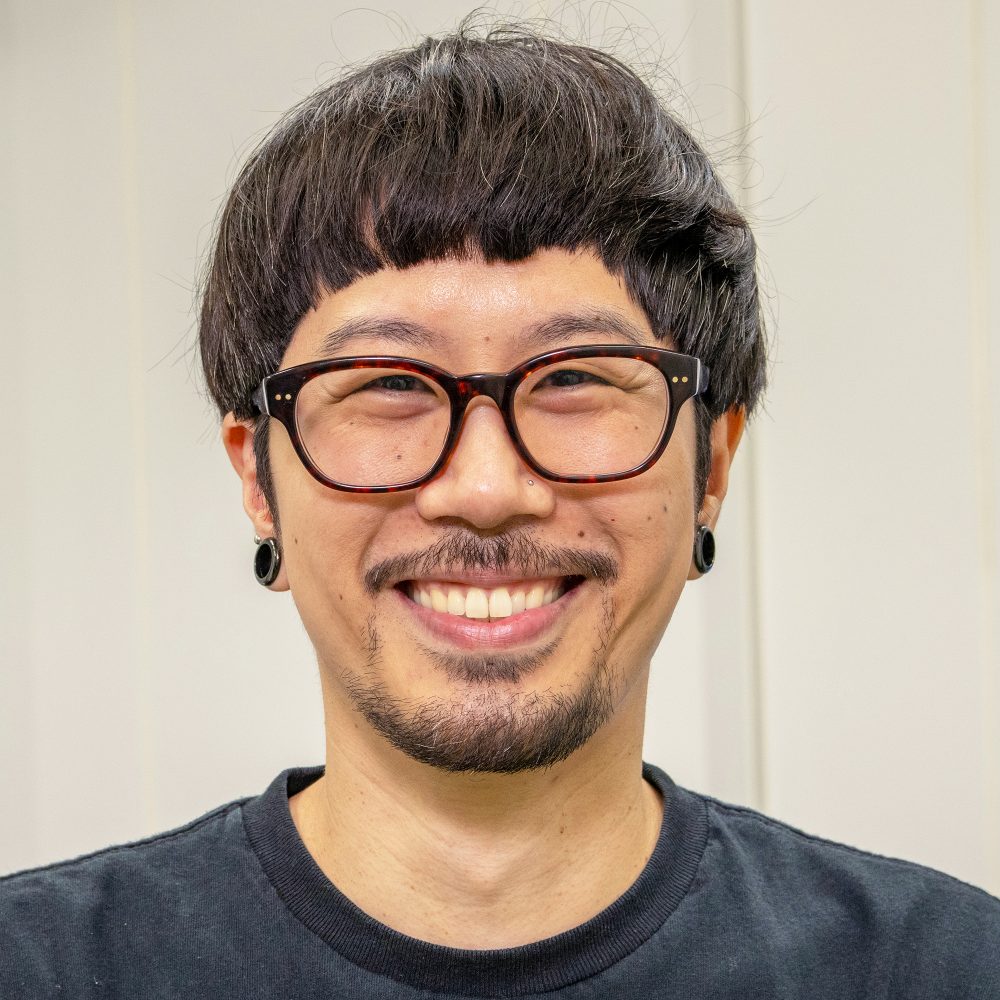
Research
The predictive coding theory proposes that the brain continuously generates and updates predictions of sensory information at multiple levels of abstraction, and emits prediction-error signals when the predicted and actual sensory inputs differ. This theory offers a comprehensive framework for understanding perception, action, and internal processes such as creativity. The current focus of my lab’s research includes:
Predictive Coding Circuits: Our goal is to unravel the complex networks of microcircuits and macrocircuits essential to predictive coding across different hierarchical levels and sensory domains. Our research spans multiple species—from rodents and marmosets to humans—and combines experimental results from these varied subjects with computational modeling to develop comprehensive insights.
Neural Markers for Prediction-Related Psychiatric Disorders: A key focus of our research is to identify mechanistic neural markers associated with psychiatric disorders characterized by prediction anomalies, such as autism spectrum disorder. Understanding these neural foundations is essential for comprehending the conditions and could lead to high-resolution diagnoses and targeted interventions.
Predictive Coding in Creativity: We also explore the role of predictive coding in creativity potential, which we define as the brain’s capacity to generate novel and useful ideas for upcoming tasks. Our goal is to provide mechanistic insights into this complex process, linking creativity directly to its neural circuit implementation.
Creativity Augmentation for Real-World Applications: One ambition of our research is to develop a closed-loop system that enhances creativity potential in practical, real-world applications. This system has the potential to transform fields that rely on creative problem-solving by providing innovative tools and methodologies to boost innovation.
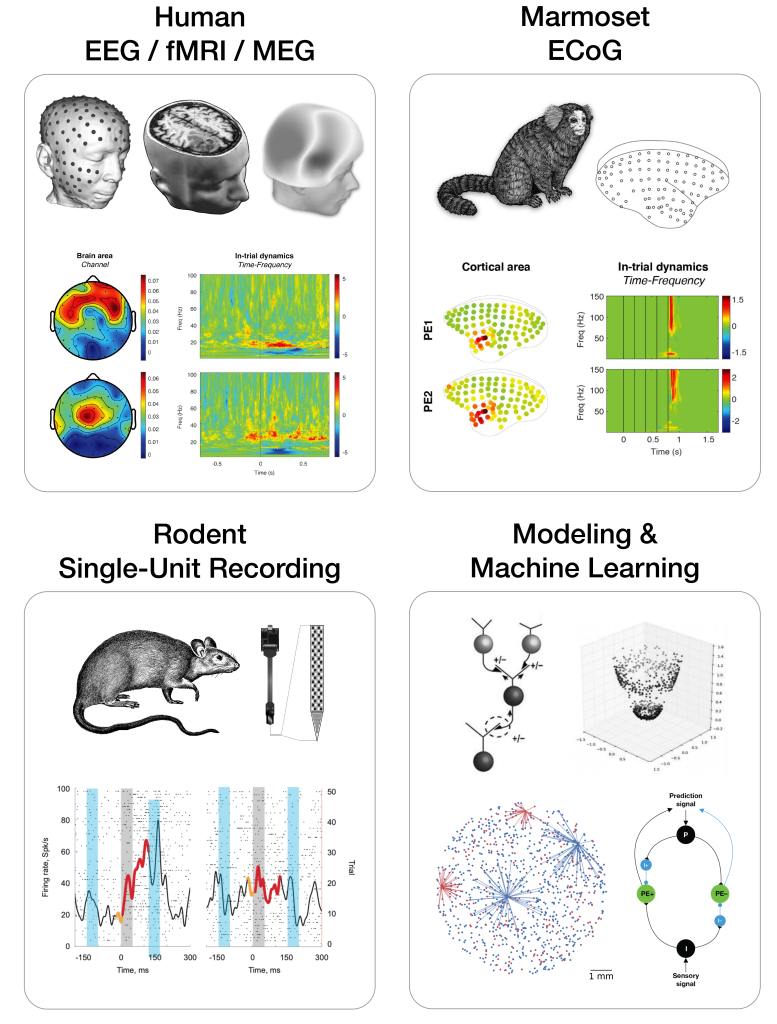
Publications
Chao ZC, Komatsu M, Matsumoto M, Iijima K, Nakagaki K, Ichinohe N (2024). Diverse configurations of erroneous predictive coding across brain hierarchies in a non-human primate model of autism spectrum disorder. Communications Biology, 7(1), 851.
Huang YT, Wu CT, Fang YM, Fu CK, Koike S, Chao ZC (2024). Crossmodal hierarchical predictive coding for audiovisual sequences in human brain. Communications Biology, 7(1), 965.
Chao ZC, Komatsu M, Matsumoto M, Iijima K, Nakagaki K, Ichinohe N (2024). Diverse configurations of erroneous predictive coding across brain hierarchies in a non-human primate model of autism spectrum disorder. Communications Biology, 7, 851.
Kern FB, Chao ZC (2023). Short-term neuronal and synaptic plasticity act in synergy for deviance detection in spiking networks. PLOS Computational Biology, 19(10), e1011554.
Chao ZC, Huang YT, Wu CT (2022). A quantitative model reveals a frequency ordering of prediction and prediction-error signals in the human brain. Communications Biology, 5(1), 1076.
Chao ZC, Takaura K, Wang L, Fujii N, Dehaene S (2018). Large-scale cortical networks for hierarchical prediction and prediction error in the primate brain. Neuron, 100(5), 1252-1266.
Chao ZC, Nagasaka Y, Fujii N (2015). Cortical network architecture for context processing in primate brain. Elife, 4, e06121.
Biography
Zenas Chao has a deep fascination with the human mind and the development of machines that mimic human intelligence. He earned B.S. degrees in Life Science and Chemistry in Taiwan before pursuing Biomedical Engineering at Georgia Institute of Technology, USA. During his Ph.D., he grew neurons in petri dishes and interfaced them with robots, demonstrating that machines equipped with an artificial organic brain can learn purposeful behaviors. Subsequently, he relocated to Japan, where he worked as a Research Scientist at the RIKEN Brain Science Institute, an Assistant Professor at the National Institute for Physiological Sciences, and a Junior Associate Professor at Kyoto University. His work in Japan focused on decoding brain signals from humans and monkeys to facilitate brain-controlled robots and computers. In 2019, Chao joined the International Research Center for Neurointelligence (IRCN) at the University of Tokyo. Here, he applies his research experience in silico, in vitro, and in vivo, to explore neural implementations of predictive coding, which is considered by many as a grand unified theory of cognition, and to investigate the neural mechanisms underlying creativity, a pivotal component of human intelligence.