Among the speed bumps on the road to a better AI, the rapid proliferation of big data has exceeded the practical processing ability of computational tools for real-world modeling. In particular, data from short time-series events with high dimensionality lack efficient and robust methods to predict future events. However, new computational frameworks may help to resolve this bottleneck to unlock a previously inaccessible range of real world applications and, potentially, the development of novel AI. In a study published by an international team of researchers from the South China University of Technology, the Chinese Academy of Sciences, and the International Research Center for Neurointelligence at The University of Tokyo, a method for real world data analysis using a modification of a class of computing algorithms called reservoir neural networks shows surprising versatility and performance.
The research team was interested in solving the problem of how to transform high dimensional, short time series data into a highly predictive neural network. They started with a framework called reservoir computing that channels the data into a “black box” reservoir with fixed dynamics and simple rules governing the input data and output performance. They modified this neural network to generate the reservoir directly from the input spatial data and use it to generate a temporal output, making the network independent of external factors and enabling use of a data processing tool called Spatiotemporal Information (STI) transformation based on delay embedding theory. They termed this framework an Auto-Reservoir Neural Network (ARNN). The ARNN can map input spatial data to future temporal values, a process called multi-step ahead prediction. Theoretical advantages of the method include improved prediction accuracy, efficiency, and robustness, from relatively small, high dimensional datasets.
The model was tested on a wide range of real world applications including data from wind speed, typhoon tracking, gene expression, stock market volatility, medical patient admissions (after air pollution epochs), and traffic speed. In each of these cases, the ARNN was able to predict future outcomes based on relatively small datasets validating the utility of the method. There are limitations to this framework including a lack of accuracy for critical transitions and in noisy systems. However, when compared to current methods like deep learning networks, the ARNN has a theoretical advantage of a simplified set of governing parameters enabling a greater computing efficiency. One of several interesting applications of ARNNs would be to use electrical brain activity and short term behavioral data from humans or animals to predict future behavior. This, in conjunction with mechanistic studies of the neurobiological meaning of “reservoirs” in living nervous systems, may improve the analysis of human intelligence.
Correspondent: Charles Yokoyama, Ph.D., IRCN Science Writing Core
Reference: Chen, P., Liu, R., Aihara, K., Chen, L. (2020) Autoreservoir Computing for Multi-Step Ahead Prediction Based on the Spatiotemporal Information Transformation. Nature Communications. Online publication: Sept. 11, 2020. DOI: 10.1038/s41467-020-18381-0
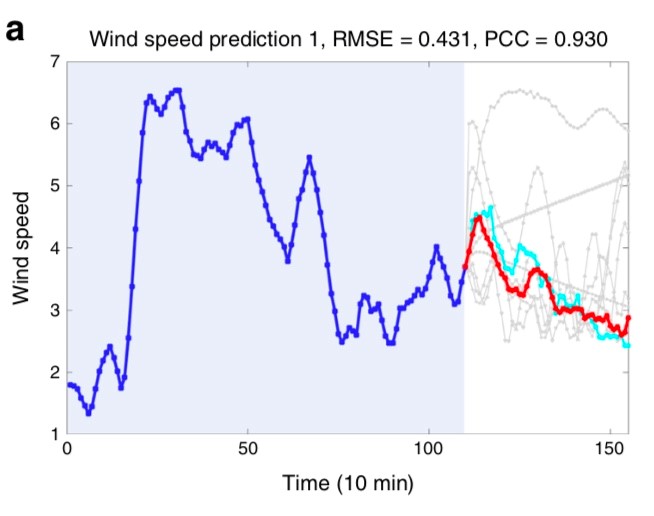
Figure: Wind speed prediction in Wakkanai, Japan (light blue trace) by an auto-reservoir neural network (ARNN) compared to other method (gray traces) where the dataset was provided by the Japan Meteorological Agency. See Reference for details.
Media Contact: The author is available for interviews in English.
University Professor Kazuyuki Aihara, Ph.D.
International Research Center for Neurointelligence (IRCN)
The University of Tokyo Institutes for Advanced Study
Mayuki Satake
Public Relations
International Research Center for Neurointelligence (IRCN)
The University of Tokyo Institutes for Advanced Study
pr@ircn.jp